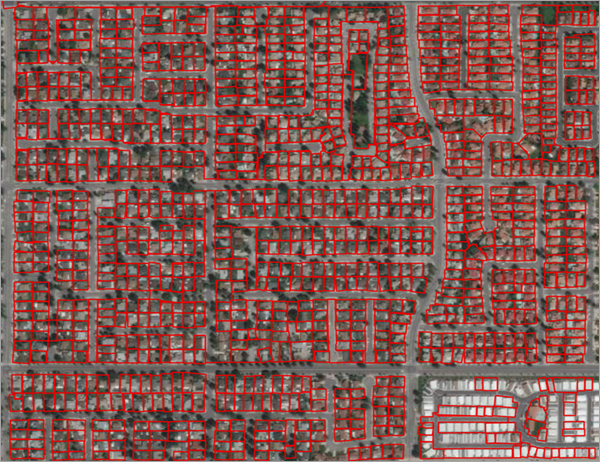
Parcels are units of land that identify property boundaries and are useful for basemap creation and for land management. Traditionally, parcel mapping has been done using highly accurate surveying techniques, but this can be expensive and time-consuming. High-resolution imagery is increasingly being used for plot delineation, and the use of deep leaning models can automate and speed up the process.
Residential parcels are often associated with visible boundaries, but since legally valid parcels can be defined without a clearly demarcated boundary, this model only deduces their plausible approximations. This model can be used to create basemaps, which can be further refined by manual editing.
Model details
This model has the following characteristics:
- Input—Raster, mosaic dataset, or image service.
- Output—Feature class containing land parcels.
- Compute—This workflow is compute intensive and a GPU with compute capability of 6.0 or higher is recommended.
- Applicable geographies—This model is designed to work well in the United States.
- Architecture—This model uses the BDCNEdgeDetector model architecture implemented in ArcGIS API for Python.
- Accuracy metrics—This model has an overall accuracy of 73.2 percent.
Access and download the model
Download the Parcel Extraction—USA pretrained model from ArcGIS Living Atlas of the World. Alternatively, access the model directly from ArcGIS Pro, or consume it in ArcGIS Online using Professional or Professional Plus user type.
- Browse to ArcGIS Living Atlas of the World.
- Sign in with your ArcGIS Online credentials.
- Search for Parcel Extraction—USA and open the item page from the search results.
- Click the Download button to download the model.
You can use the downloaded .dlpk file directly in ArcGIS Pro, or upload and use it in ArcGIS Enterprise. Additionally, you can fine-tune the pretrained model if necessary.
Release notes
The following are the release notes:
Date | Description |
---|---|
July 2021 |
|