One of the most commons reasons to use recurring big data analytics is to perform processing in near real-time. For example, you can configure a big data analytic to run every few minutes or hours that processes only the most recent features written and stored in a feature layer.
As another example, consider a real-time analytic configured to receive data from a feed that collects vehicle location updates every 10 seconds. This real-time analytic writes event data to a Feature Layer (new) output and calculates a date field (named something such as process_timestamp) using the Calculate Field tool with the time in which an event was processed using the Arcade Date() function.
Note:
It is a best practice to use the Calculate Field tool in a real-time analytic to write the date and time of processing to the feature layer that will be consumed by the big data analytic for near real-time analysis. Some data sources consumed by feeds have an inherent delay in providing data or polling that could cause features to be missed by the timestamp field queries.
To complement this real-time analytic, a scheduled recurring big data analytic can be configured that uses the output of the real-time analytic as its data source. In this recurring big data analytic, a Feature Layer source is configured to collect the feature layer output created by the real-time analytic. When configuring a Feature Layer source, in the Timestamp Field step, a date field can be selected in the Date field for latest features parameter. Select the date and time field created by the Calculate Field tool in the real-time analytic. In this example, the field name is process_timestamp.
The Feature Layer source uses the timestamp value to retrieve only the latest features from the feature layer on each run. If a field is selected for the Date field for latest features parameter, the first time ArcGIS Velocity polls the feature layer, it will load all features with a timestamp date and time less than the first scheduled run time that also meets the criteria of the WHERE clause. With each subsequent run, features with a timestamp value between the last scheduled run time and the current scheduled run time that also meet the criteria of the WHERE clause will be loaded.
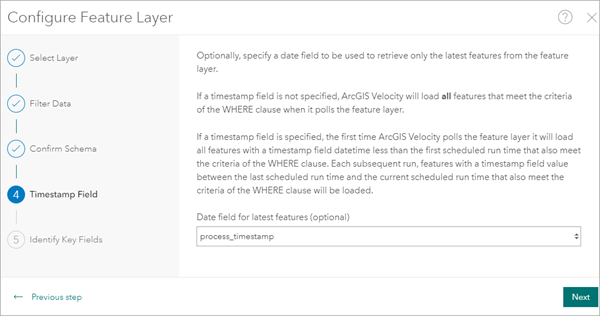
The big data analytic is configured to run at the desired repeat interval such as every 5 minutes. Using a timestamp field as outlined above, only the most recent features not yet processed are analyzed by the big data analytic during subsequent runs.