The Average Nearest Neighbor tool measures the distance between each feature centroid and its nearest neighbor's centroid location. It then averages all these nearest neighbor distances. If the average distance is less than the average for a hypothetical random distribution, the distribution of the features being analyzed is considered clustered. If the average distance is greater than a hypothetical random distribution, the features are considered dispersed. The average nearest neighbor ratio is calculated as the observed average distance divided by the expected average distance (with expected average distance being based on a hypothetical random distribution with the same number of features covering the same total area).
Calculations
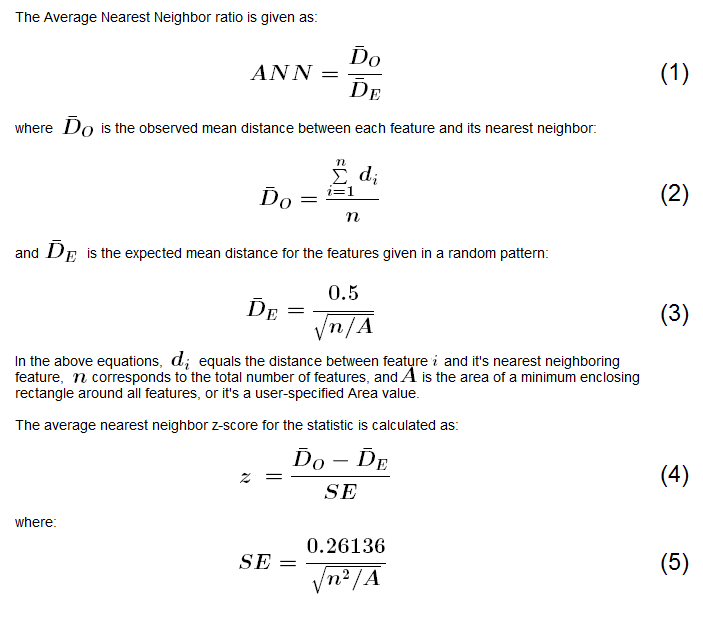
Interpretation
If the index (average nearest neighbor ratio) is less than 1, the pattern exhibits clustering. If the index is greater than 1, the trend is toward dispersion.
The equations used to calculate the average nearest neighbor distance index (1) and z-score (4) are based on the assumption that the points being measured are free to locate anywhere within the study area (for example, there are no barriers, and all cases or features are located independently of one another). The p-value is a numerical approximation of the area under the curve for a known distribution, limited by the test statistic. See What is a z-score? What is a p-value? for more information about these statistics.
Caution:
The z-score and p-value for this statistic are sensitive to changes in the study area or changes to the Area parameter. For this reason, only compare z-score and p-value results from this statistic when the study area is fixed.
Output
Possible applications
- Evaluate competition or territory: Quantify and compare the spatial distribution of a variety of plant or animal species within a fixed study area; compare average nearest neighbor distances for different types of businesses within a city.
- Monitor changes over time: Evaluate changes in spatial clustering for a single type of business within a fixed study area over time.
- Compare an observed distribution to a control distribution: In a timber analysis, you may want to compare the pattern of harvested areas to the pattern of harvestable areas to determine if cut areas are more clustered than you would expect, given the distribution of harvestable timber overall.
Additional resources
The following books have further information about this tool:
Ebdon, David. Statistics in Geography. Blackwell, 1985.
Mitchell, Andy. The ESRI Guide to GIS Analysis, Volume 2. ESRI Press, 2005.